Author: Harmeet Singh Bagga
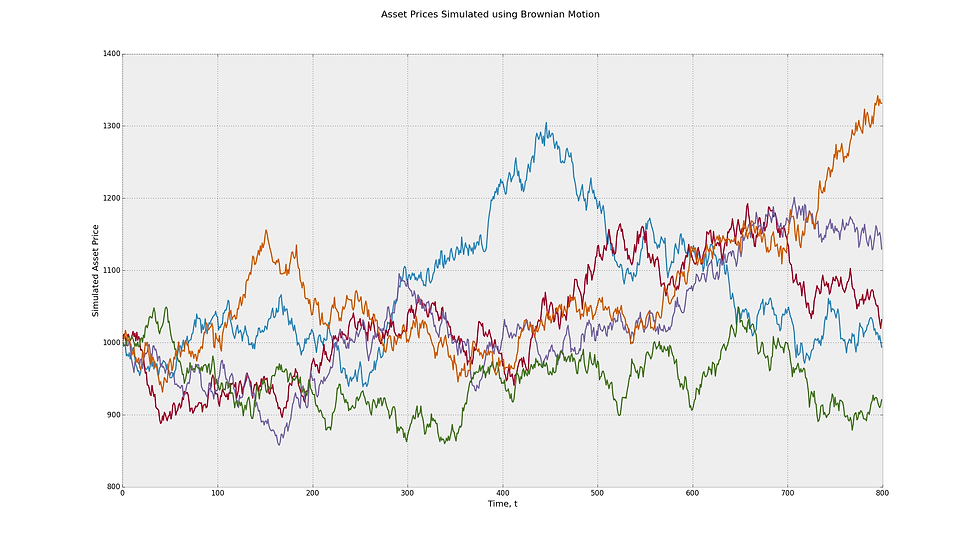
In probability theory and related fields, a stochastic or random process is a mathematical object usually defined as a collection of random variables. A stochastic process can also be understood as a description of the movement of an object over time or a set of ordered random variables, where the order is defined over time. At every new unit of time, the object can assume one of many possible positions, and each position has a probability associated with it. The exact path that the object will take is unknown, though we can make inferences about the path it might take based those probabilities.
The set of different possible positions or values that a stochastic variable may take is called its 'State Space' and most often, these stochastic variables are studied over a period of time, the set of time positions for each random variable is called its 'Time Domain'.
Both the State Space and the Time Domain of a stochastic process can be discrete or continuous. And henceforth, on this basis the stochastic processes can be classified into 4 major categories. The class of Stochastic Processes with Discrete State Space and a Discrete Time Domain, which satisfy the Markov Property are called the 'Markov Chains'. Markov Property of a Stochastic Process means that the future prediction about the value/position of the random variable depends only its current value/position and not on any past value or positions assumed by the stochastic variable. This conditional independence from past data or memoryless-ness on part of Stochastic Variables forms an important class of them.
In general, any stochastic process can be defined in terms of its value at different times or increments in its value at different times.
This forms the base for many important properties of a Stochastic Process, namely its Increments, Filterations, etc. Based on these properties, we can classify the elements of State Space into various categories like absorbing states, non-absorbing states etc.
Any Markov Chain can be represented in 3 ways; the Algebraic way, via Transition Diagrams or via Transition Matrix. The Transition Diagram is a visual representation of all the pathways a stochastic variable may take over time and the probabilities associated with each pathway. These diagrams if drawn for one period ahead modelling, are called One-Step Transition Diagrams and if drawn for any n>1 then are called n-Step Transition Diagrams and correspondingly, we can derive the one-step of n-step ahead Transition Probabilities.
Due to the ease of representation and interpretation, the Markov Chain Models are applicable in many real-life scenarios like the HSD (Healthy-Sick-Dead) Model in life Insurance Industry, The NCD (No-Claim-Discount) Model in the Motor Insurance. This technique is widely applicable in finance industry to show the movement of stocks, in meteorological departments to show weather transitions, for demographic studies of population movements across regions to name a few.
Comments